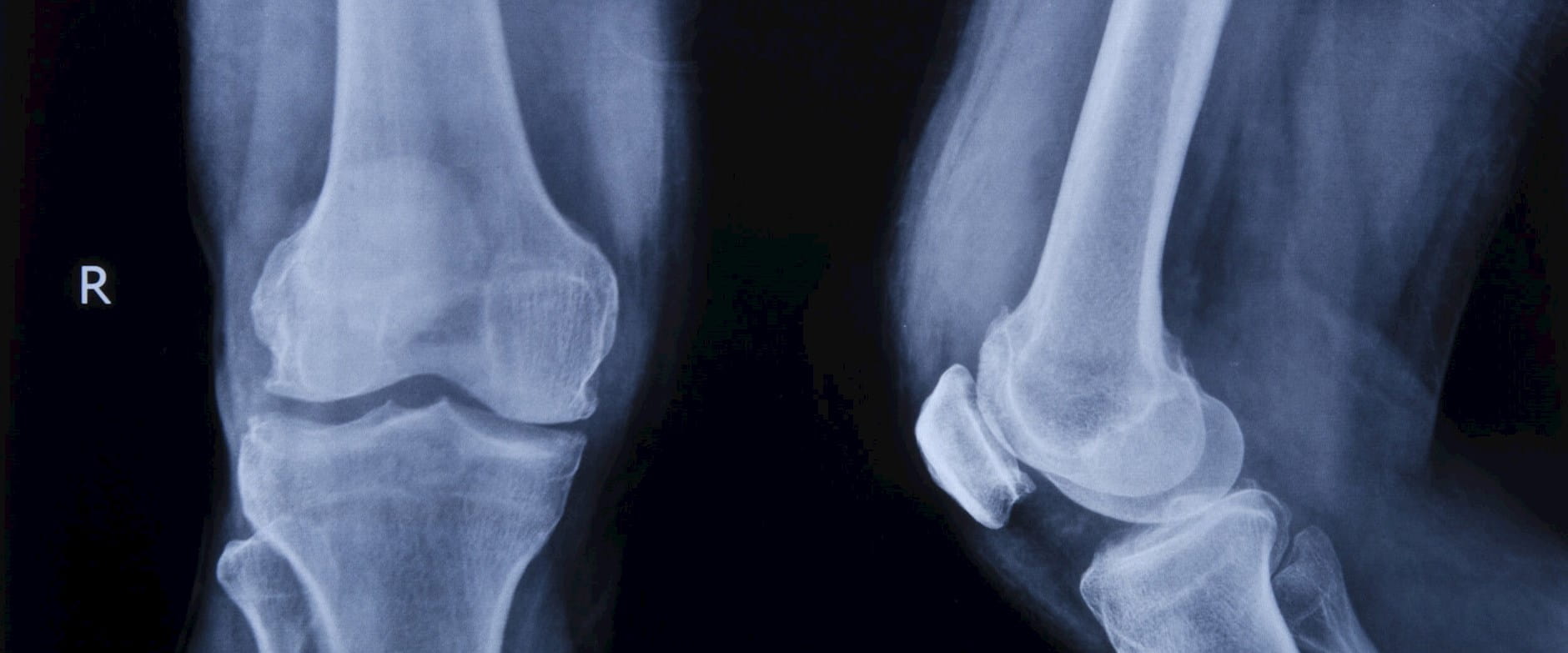
Reducing Racial Disparities in Knee Pain using Artificial Intelligence
Reducing Racial Disparities in Knee Pain using Artificial Intelligence
Senthil Nachimuthu, MD, PhD
Nightingale Open Science, CAAI, Chicago Booth
Reducing health disparities using artificial intelligence is a key focus area for the Center for Applied Artificial Intelligence. The Center has recently done research in using AI to address the racial disparities associated with knee pain. Studies have found that a higher proportion of African American patients experience knee pain with higher severity compared to the general population. In addition, patients with incomes that are less than $50,000/year or those without a college education have higher prevalence and severity of knee pain. Many of them are not diagnosed as having osteoarthritis from their X-rays using the current standards of care. Instead, their knee pain is often attributed to causes external to the knee, such as life stress, social isolation, etc. However, these psychosocial factors only explain 9% of pain disparities in African American patients.
In a 2021 study published in Nature, Dr. Emma Pierson (Cornell Tech), along with Dr. Sendhil Mullainathan (UChicago), Dr. Ziad Obermeyer (UC Berkeley) and others, demonstrated that applying artificial intelligence to knee X-ray images can identify new features within the knee joint that explain the disparities of knee pain in these patients. [1] This algorithm explained pain disparities in about 43% of African American patients who are not diagnosed as having osteoarthritis using KLG score. KLG Score is the standard used to diagnose osteoarthritis in knee X-rays, which was created from clinical studies on white British patients in the 1950s. [2] This score might miss physical causes of pain in non-white patients. After clinical validation, the new hypotheses discovered by applying artificial intelligence can help diagnose and treat patients who were previously underrepresented in clinical studies in more accurate and timely manners.
Research and development of new algorithms for medical diagnosis and treatment requires vast amounts of data. However, medical data sets needed for machine learning research are not widely available. They are siloed at medical universities and research institutes due to patient privacy regulations and institutional data sharing restrictions. Hence, the data are not available to researchers outside these institutes. Researchers within these institutes need to meet stringent and time-consuming requirements to obtain access to data as well. In addition, computational platforms for AI research are expensive and are not widely available to all researchers.
Nightingale Open Science was founded at the Center for Applied Artificial Intelligence to open data access to academic researchers worldwide. Nightingale also provides a centralized compute platform so that researchers can conduct machine learning research without having large computing infrastructure of their own. To date, Nightingale has published five large medical data sets including the world’s largest open data set of cancer biopsy slides. This breast cancer biopsy data set was contributed by Providence Oncology. Other data sets related to conditions such as COVID-19, chest fractures and heart attacks were contributed by Providence St. Joseph Health, Stanford Medicine, Contra Costa County Health System, and National Taiwan University. More than 500 researchers from around the world have registered on the Nightingale platform to conduct research using these data sets, which would not have been possible otherwise.
The algorithm to explain knee pain disparities was created by Dr. Emma Pierson and others using X-rays and other data of about 4,200 patients included in the Osteoarthritis Initiative data set published by the National Institutes of Health. [3] About 17% of patients in this data set were African American when this study was performed. A need for a much larger data set to validate and refine such algorithms on a larger and more diverse population has been well recognized.
To replicate this study on a much larger and diverse population, and to make this data set available to researchers worldwide along with the computational infrastructure required to conduct such research, Nightingale has started working with clinical researchers at a leading university in the United States. Nightingale has received a grant from Lacuna Fund to support the aggregation, deidentification, manual annotation by clinicians, and publication on the Nightingale Platform. This data set will include the knee X-rays, demographics, and clinical outcomes of tens of thousands of patients. We will describe our partnerships and efforts that enable this data set in a future publication.
The data set is expected to be published in 2023. This data set will enable machine learning researchers worldwide to discover new knowledge about signs in knees that are associated with knee pain and may lead to new knowledge being added to the body of medical knowledge after clinical validation.
References
[1] Pierson E, Cutler DM, Leskovec J, Mullainathan S, Obermeyer Z. An algorithmic approach to reducing unexplained pain disparities in underserved populations. Nature Medicine. 2021 Jan;27(1):136-40. Link: https://www.nature.com/articles/s41591-020-01192-7
[2] Kellgren, J. H. & Lawrence, J. S. Radiological assessment of osteo-arthrosis.
Ann. Rheum. Dis. 16, 494–502 (1957). Link: http://dx.doi.org/10.1136/ard.16.4.494
[3] Nevitt, M. C., Felson, D. T. & Lester, G. Te Osteoarthritis Initiative.
https://nda.nih.gov/oai/ (2006).