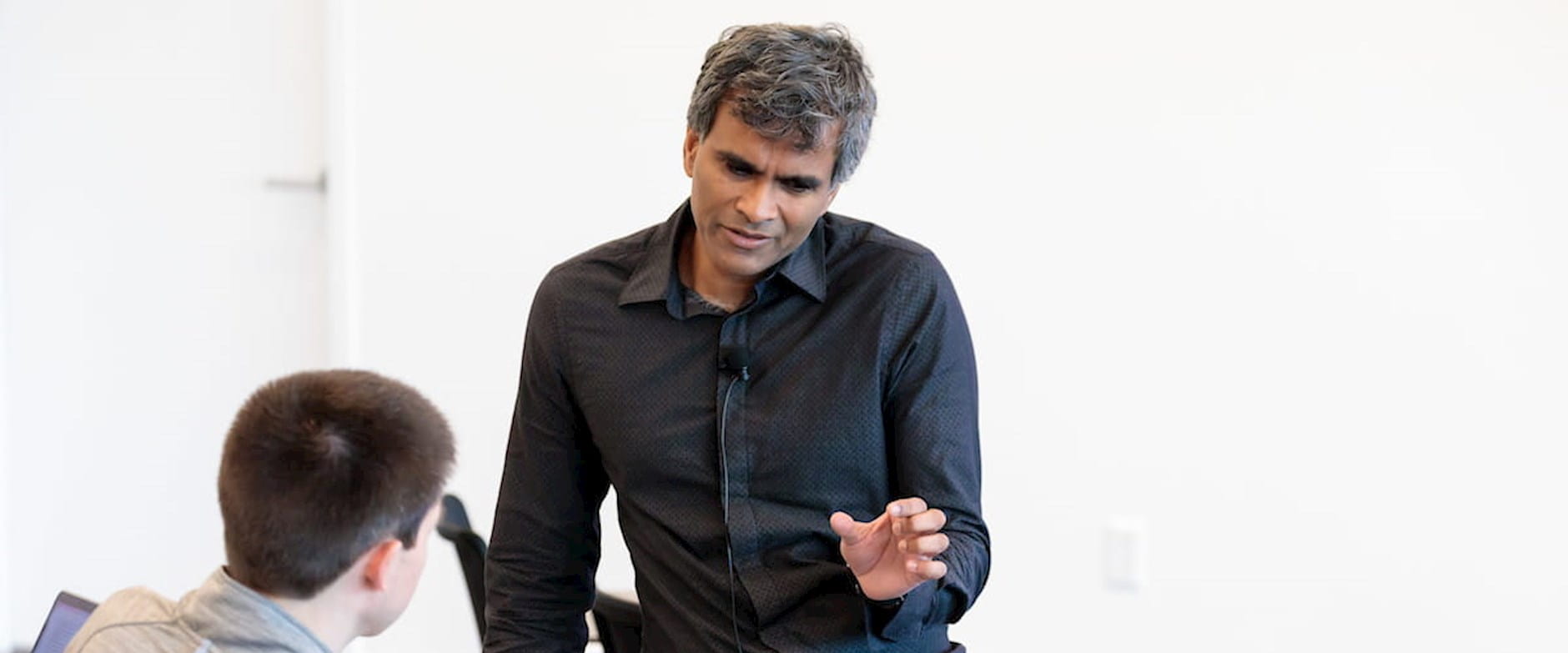
Artificial Intelligence Workshop
Workshop Details
This workshop invites faculty and guests to present their current research within applied artificial intelligence.
Please contact Abigail Scott if you would like to be added to the listserv for weekly updates.
Spring Workshop Series
March 25 | 10-11:20am | C04
Dilip Arumugam
Stanford University
Title: Deciding What to Learn
Abstract: The traditional literature on balancing exploration and exploitation focuses on environments in which an agent can approach optimal performance within a relevant time frame. However, modern artificial decision-making agents engage with complex environments – such as the World Wide Web – in which there is no hope of approaching optimal performance within any relevant time frame. In such environments, rather than endeavor to obtain enough information salient to optimal behavior, an agent should instead target a modest corpus of information that, while capable of facilitating behavioral improvement, is itself insufficient to enable near-optimal performance. We design an agent that modulates exploration in this way and provide both a theoretical as well as an empirical analysis of its behavior. In effect, at each time, this agent decides what to learn so as to achieve a desired trade-off between information requirements and performance.
March 27 | 5-6:20pm | C05
Kawin Ethayarajh
Stanford University
Title: Machine Learning under Real-World Incentives
Abstract: We have long accepted that machine learning is bottlenecked by what hardware and software can do; we often discuss whether a process is memory-bound or compute-bound, for example. In this talk, I propose that machine learning is also incentive-bound: i.e., what it can accomplish is often determined by the incentives of real-world actors such as workers, firms, and states. These incentives are often unstated, hard to change, and sometimes in conflict with one another. My work formalizes these incentives so that we can build machine learning pipelines that work as well in the real world as they do on paper. In this talk, I will discuss: (1) how to overcome conflicting incentives to create datasets as complex as real-world problems; (2) how prospect theory can enable us to do pluralistic model alignment instead of imposing one set of values on everyone; (3) how to do cost-sensitive evaluation that better captures how people choose among models in the real world. Artefacts of this research -- such as the Stanford Human Preferences (SHP) dataset, Kahneman-Tversky Optimization (KTO), and Dynaboard --have been widely adopted in both academia and industry.
April 9 | 5-6:20pm | C05
Sarah Cen
Massachusetts Institute of Technology
Title: Paths to AI Accountability
Abstract: We have begun grappling with difficult questions related to the rise of AI, including: What rights do individuals have in the age of AI? When should we regulate AI and when should we abstain? What degree of transparency is needed to monitor AI systems? These questions are all concerned with AI accountability: determining who owes responsibility and to whom in the age of AI. In this talk, I will discuss the two main components of AI accountability, then illustrate them through a case study on social media. Within the context of social media, I will focus on how social media platforms filter (or curate) the content that users see. I will review several methods for auditing social media, drawing from concepts and tools in hypothesis testing, causal inference, and LLMs.
April 11 | 5-6:20pm | C04
Theodore Sumers
Princeton University
Abstract forthcoming