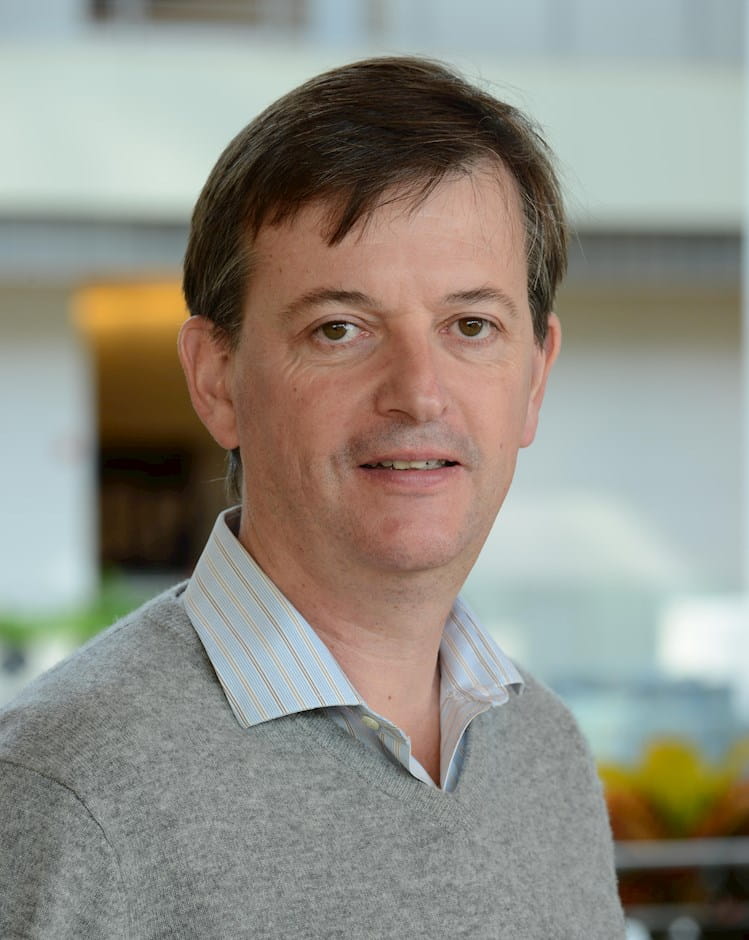
Nicholas Polson
Robert Law, Jr. Professor of Econometrics and Statistics
Robert Law, Jr. Professor of Econometrics and Statistics
Nicholas Polson is a Bayesian statistician who conducts research on Financial Econometrics, Markov chain Monte Carlo, Particle learning, and Bayesian inference. Inspired by an interest in probability, Polson has developed a number of new algorithms and applied them to the fields of statistics and financial econometrics, including the Bayesian analysis of stochastic volatility models and sequential particle learning for statistical inference.
Polson’s article, “Bayesian Analysis of Stochastic Volatility Models,” was named one of the most influential articles in the 20th anniversary issue of the Journal of Business and Economic Statistics. His recent work includes methods for sparse Bayesian estimation with application to high dimensional regression and classification.
With M. Johannes, "MCMC Methods for Financial Econometrics," Handbook of Financial Econonmetrics (2004).
With B. Eraker and M. Johannes, "The Impact of Jumps in Volatility in Returns," Journal of Finance (2003).
With E. Jacquier and P. Rossi, "Bayesian Analysis of Stochastic Volatility Models," Journal of Business and Economic Statistics (1994, 2002).
Invited paper with discussion, "Convergence of Markov Chain Monte Carlo Algorithms," Fifth Valencia Meeting on Bayesian Statistics.
For a listing of research publications, please visit the university library listing page.
REVISION: Deep Learning in Characteristics-Sorted Factor Models
Date Posted:Mon, 22 Feb 2021 04:01:53 -0600
To study the characteristics-sorted factor model in empirical asset pricing, we design a non-reduced-form feedforward neural network with the non-arbitrage objective to minimize pricing errors. Our model starts from firm characteristics [inputs], generates risk factors [intermediate features], and fits the cross-sectional returns [outputs]. A nonlinear activation in deep learning approximates the traditional security sorting on characteristics to create long-short portfolio weights, like a hidden layer, from lag characteristics to realized returns. Our model offers an alternative approach for dimension reduction in empirical asset pricing on characteristics [inputs], rather than factors [intermediate features], and allows for both nonlinearity and interactions directly through [inputs]. Our empirical findings are threefold. First, we find substantial and robust asset pricing improvements of multiple performance measures, such as Cross-Sectional R^2, in both in-sample and ...
REVISION: Deep Learning in Characteristics-Sorted Factor Models
Date Posted:Wed, 25 Mar 2020 04:51:24 -0500
To study the characteristics-sorted factor model in asset pricing, we develop a bottom-up approach with state-of-the-art deep learning optimization. With an economic objective to minimize pricing errors, we train a non-reduced-form neural network using firm characteristics [inputs], and generate factors [intermediate features], to fit security returns [outputs]. Sorting securities on firm characteristics provides a nonlinear activation to create long-short portfolio weights, as a hidden layer, from lag characteristics to realized returns. Our model offers an alternative perspective for dimension reduction on firm characteristics [inputs], rather than factors [intermediate features], and allows for both nonlinearity and interactions on inputs. Our empirical findings are twofold. We find robust statistical and economic evidence in evaluating various portfolios and individual stock returns. Finally, we show highly significant results in factor investing, improvement in dissecting ...
REVISION: Deep Learning in Characteristics-Sorted Factor Models
Date Posted:Fri, 01 Nov 2019 03:55:51 -0500
To study the characteristics-sorted factor model in asset pricing, we develop a bottom-up approach with state-of-the-art deep learning optimization. With an economic objective to minimize pricing errors, we train a non-reduced-form neural network using firm characteristics [inputs], and generate factors [intermediate features], to fit security returns [outputs]. Sorting securities on firm characteristics provides a nonlinear activation to create long-short portfolio weights, as a hidden layer, from lag characteristics to realized returns. Our model offers an alternative perspective for dimension reduction on firm characteristics [inputs], rather than factors [intermediate features], and allows for both nonlinearity and interactions on inputs. Our empirical findings are twofold. We find robust statistical and economic evidence in out-of-sample portfolios and individual stock returns. To interpret our deep factors, we show highly significant results in factor investing via the squared ...
REVISION: Deep Learning in Characteristics-Sorted Factor Models
Date Posted:Mon, 07 Oct 2019 05:33:23 -0500
To study the characteristics-sorted factor model in asset pricing, we develop a bottom-up approach with state-of-the-art deep learning optimization. With an economic objective to minimize pricing errors, we train a non-reduced-form neural network using firm characteristics [inputs], and generate factors [intermediate features], to fit security returns [outputs]. Sorting securities on firm characteristics provides a nonlinear activation to create long-short portfolio weights, as a hidden layer, from lag characteristics to realized returns. Our model offers an alternative perspective for dimension reduction on firm characteristics [inputs], rather than factors [intermediate features], and allows for both nonlinearity and interactions on inputs. Our empirical findings are twofold. We find robust statistical and economic evidence in "unseen" portfolios and individual stock returns. To interpret our deep factors, we show strong improvement in investing factors as well as dissecting ...
REVISION: Deep Learning for Finance: Deep Portfolios
Date Posted:Mon, 29 Apr 2019 03:01:33 -0500
We explore the use of deep learning hierarchical models for problems in financial prediction and classification. Financial prediction problems – such as those presented in designing and pricing securities, constructing portfolios, and risk management – often involve large data sets with complex data interactions that currently are difficult or impossible to specify in a full economic model. Applying deep learning methods to these problems can produce more useful results than standard methods in finance. In particular, deep learning can detect and exploit interactions in the data that are, at least currently, invisible to any existing financial economic theory.
REVISION: Why Indexing Works
Date Posted:Mon, 29 Apr 2019 03:01:20 -0500
We develop a simple stock selection model to explain why active equity managers tend to underperform a benchmark index. We motivate our model with the empirical observation that the best performing stocks in a broad market index often perform much better than the other stocks in the index. Randomly selecting a subset of securities from the index may dramatically increase the chance of underperforming the index. The relative likelihood of underperformance by investors choosing active management likely is much more important than the loss to those same investors from the higher fees for active management relative to passive index investing. Thus, active management may be even more challenging than previously believed, and the stakes for finding the best active managers may be larger than previously assumed.
REVISION: Deep Learning for Finance: Deep Portfolios
Date Posted:Sun, 17 Feb 2019 16:21:58 -0600
We explore the use of deep learning hierarchical models for problems in financial prediction and classification. Financial prediction problems – such as those presented in designing and pricing securities, constructing portfolios, and risk management – often involve large data sets with complex data interactions that currently are difficult or impossible to specify in a full economic model. Applying deep learning methods to these problems can produce more useful results than standard methods in finance. In particular, deep learning can detect and exploit interactions in the data that are, at least currently, invisible to any existing financial economic theory.
REVISION: Why Indexing Works
Date Posted:Sun, 17 Feb 2019 16:04:47 -0600
We develop a simple stock selection model to explain why active equity managers tend to underperform a benchmark index. We motivate our model with the empirical observation that the best performing stocks in a broad market index often perform much better than the other stocks in the index. Randomly selecting a subset of securities from the index may dramatically increase the chance of underperforming the index. The relative likelihood of underperformance by investors choosing active management likely is much more important than the loss to those same investors from the higher fees for active management relative to passive index investing. Thus, active management may be even more challenging than previously believed, and the stakes for finding the best active managers may be larger than previously assumed.
REVISION: Deep Learning in Asset Pricing
Date Posted:Fri, 08 Feb 2019 16:38:56 -0600
Deep learning provides a framework for characteristics-based factor modeling in empirical asset pricing. We provide a systematic approach for long-short factor generation with a goal to minimize pricing errors in the cross section. Security sorting on firm characteristics provides a nonlinear activation function as part of a deep learning model. Our deep factors are tradable and allow for both nonlinearity and interactions between predictors. For cross-sectional return prediction, we study monthly U.S. equity returns based on lag firm characteristics and macro predictors from 1975 to 2017 with a universe of 3,000 stocks. Finally, with additional deep factors, we find the out-of-sample forecast improvements for anomaly-sorted and industry portfolios.
Update: Deep Learning for Finance: Deep Portfolios
Date Posted:Sat, 01 Dec 2018 07:31:55 -0600
We explore the use of deep learning hierarchical models for problems in financial prediction and classification. Financial prediction problems – such as those presented in designing and pricing securities, constructing portfolios, and risk management – often involve large data sets with complex data interactions that currently are difficult or impossible to specify in a full economic model. Applying deep learning methods to these problems can produce more useful results than standard methods in finance. In particular, deep learning can detect and exploit interactions in the data that are, at least currently, invisible to any existing financial economic theory.
The Paper was removed
Update: Why Indexing Works
Date Posted:Sat, 01 Dec 2018 07:31:46 -0600
We develop a simple stock selection model to explain why active equity managers tend to underperform a benchmark index. We motivate our model with the empirical observation that the best performing stocks in a broad market index often perform much better than the other stocks in the index. Randomly selecting a subset of securities from the index may dramatically increase the chance of underperforming the index. The relative likelihood of underperformance by investors choosing active management likely is much more important than the loss to those same investors from the higher fees for active management relative to passive index investing. Thus, active management may be even more challenging than previously believed, and the stakes for finding the best active managers may be larger than previously assumed.
The Paper was removed
REVISION: Deep Learning Factor Alpha
Date Posted:Mon, 01 Oct 2018 05:37:33 -0500
Does a factor model exist to absorb all existing anomalies? We provide a deep learning automated solution to generate long-short factors using a high-dimensional firm characteristic. Sorting securities on firm characteristics is a common practice in finance and a nonlinear activation function built into deep learning. Our algorithm performs a nonlinear search and finds the optimal transformation of characteristics used for security sorting, with one asset pricing objective: minimizing alphas. Our deep factors, hidden neurons in the neural network, are trained greedily with the backward propagation feedback from the loss function that considers both time series and cross-sectional variations. Our conditional forecast generalizes a benchmark, such as CAPM, and includes Fama-French type models as special cases. We have designed a train-validation-test study for monthly U.S. equity returns from 1975 to 2017 and 57 published firm characteristics. In an out-of-sample evaluation, the ...
REVISION: Deep Learning Factor Alpha
Date Posted:Sun, 23 Sep 2018 06:43:27 -0500
Does a factor model exist to absorb all existing anomalies? We provide a deep learning automated solution to generate long-short factors using a high-dimensional firm characteristic. Sorting securities on firm characteristics is a common practice in finance and a nonlinear activation function built into deep learning. Our algorithm performs a nonlinear search and finds the optimal transformation of characteristics used for security sorting, with one asset pricing objective: minimizing alphas. Our deep factors, hidden neurons in the neural network, are trained greedily with the backward propagation feedback from the loss function that considers both time series and cross-sectional variations. Our conditional forecast generalizes a benchmark, such as CAPM, and includes Fama-French type models as special cases. We have designed a train-validation-test study for monthly U.S. equity returns from 1975 to 2017 and 57 published firm characteristics. In an out-of-sample evaluation, the ...
REVISION: Why Indexing Works
Date Posted:Thu, 15 Mar 2018 14:20:00 -0500
We develop a simple stock selection model to explain why active equity managers tend to underperform a benchmark index. We motivate our model with the empirical observation that the best performing stocks in a broad market index often perform much better than the other stocks in the index. Randomly selecting a subset of securities from the index may dramatically increase the chance of underperforming the index. The relative likelihood of underperformance by investors choosing active management likely is much more important than the loss to those same investors from the higher fees for active management relative to passive index investing. Thus, active management may be even more challenging than previously believed, and the stakes for finding the best active managers may be larger than previously assumed.
REVISION: Deep Learning for Finance: Deep Portfolios
Date Posted:Thu, 15 Mar 2018 13:41:03 -0500
We explore the use of deep learning hierarchical models for problems in financial prediction and classification. Financial prediction problems – such as those presented in designing and pricing securities, constructing portfolios, and risk management – often involve large data sets with complex data interactions that currently are difficult or impossible to specify in a full economic model. Applying deep learning methods to these problems can produce more useful results than standard methods in finance. In particular, deep learning can detect and exploit interactions in the data that are, at least currently, invisible to any existing financial economic theory.
Update: Why Indexing Works
Date Posted:Thu, 08 Mar 2018 15:07:41 -0600
We develop a simple stock selection model to explain why active equity managers tend to underperform a benchmark index. We motivate our model with the empirical observation that the best performing stocks in a broad market index often perform much better than the other stocks in the index. Randomly selecting a subset of securities from the index may dramatically increase the chance of underperforming the index. The relative likelihood of underperformance by investors choosing active management likely is much more important than the loss to those same investors from the higher fees for active management relative to passive index investing. Thus, active management may be even more challenging than previously believed, and the stakes for finding the best active managers may be larger than previously assumed.
The Paper was removed
Update: Deep Learning for Finance: Deep Portfolios
Date Posted:Thu, 08 Mar 2018 15:07:32 -0600
We explore the use of deep learning hierarchical models for problems in financial prediction and classification. Financial prediction problems – such as those presented in designing and pricing securities, constructing portfolios, and risk management – often involve large data sets with complex data interactions that currently are difficult or impossible to specify in a full economic model. Applying deep learning methods to these problems can produce more useful results than standard methods in finance. In particular, deep learning can detect and exploit interactions in the data that are, at least currently, invisible to any existing financial economic theory.
The Paper was removed
REVISION: Sparse Regularization in Marketing and Economics
Date Posted:Sat, 10 Feb 2018 10:15:14 -0600
Sparse alpha-norm regularization has many data-rich applications in Marketing and Economics. Alpha-norm, in contrast to lasso and ridge regularization, jumps to a sparse solution. This feature is attractive for ultra high-dimensional problems that occur in demand estimation and forecasting. The alpha-norm objective is nonconvex and requires coordinate descent and proximal operators to find the sparse solution. We study a typical marketing demand forecasting problem, grocery store sales for salty snacks, that has many dummy variables as controls. The key predictors of demand include price, equivalized volume, promotion, flavor, scent, and brand effects. By comparing with many commonly used machine learning methods, alpha-norm regularization achieves its goal of providing accurate out-of-sample estimates for the promotion lift effects. Finally, we conclude with directions for future research.
REVISION: Sparse Regularization in Marketing and Economics
Date Posted:Mon, 05 Feb 2018 19:43:57 -0600
Sparse alpha-norm regularization has many data-rich applications in Marketing and Economics. Alpha-norm, in contrast to lasso and ridge regularization, jumps to a sparse solution. This feature is attractive for ultra high-dimensional problems that occur in demand estimation and forecasting. The alpha-norm objective is nonconvex and requires coordinate descent and proximal operators to find the sparse solution. We study a typical marketing demand forecasting problem, grocery store sales for salty snacks, that has many dummy variables as controls. The key predictors of demand include price, equivalized volume, promotion, flavor, scent, and brand effects. By comparing with many commonly used machine learning methods, alpha-norm regularization achieves its goal of providing accurate out-of-sample estimates for the promotion lift effects. Finally, we conclude with directions for future research.
REVISION: Deep Learning for Finance: Deep Portfolios
Date Posted:Sun, 07 Jan 2018 23:16:52 -0600
We explore the use of deep learning hierarchical models for problems in financial prediction and classification. Financial prediction problems – such as those presented in designing and pricing securities, constructing portfolios, and risk management – often involve large data sets with complex data interactions that currently are difficult or impossible to specify in a full economic model. Applying deep learning methods to these problems can produce more useful results than standard methods in finance. In particular, deep learning can detect and exploit interactions in the data that are, at least currently, invisible to any existing financial economic theory.
REVISION: Why Indexing Works
Date Posted:Sun, 07 Jan 2018 23:16:14 -0600
We develop a simple stock selection model to explain why active equity managers tend to underperform a benchmark index. We motivate our model with the empirical observation that the best performing stocks in a broad market index often perform much better than the other stocks in the index. Randomly selecting a subset of securities from the index may dramatically increase the chance of underperforming the index. The relative likelihood of underperformance by investors choosing active management likely is much more important than the loss to those same investors from the higher fees for active management relative to passive index investing. Thus, active management may be even more challenging than previously believed, and the stakes for finding the best active managers may be larger than previously assumed.
REVISION: Sparse Regularization in Marketing and Economics
Date Posted:Mon, 21 Aug 2017 02:31:44 -0500
Sparse alpha-norm regularization has many data-rich applications in marketing and economics. In contrast to traditional lasso and ridge regularization, the alpha-norm penalty has the property of jumping to a sparse solution. This is an attractive feature for ultra high-dimensional problems that occur in market demand estimation and forecasting. The underlying nonconvex regularization problem is solved via coordinate descent, and a proximal operator. To illustrate our methodology, we study a classic demand forecasting problem of Bajari, Nekipelov, Ryan, and Yang (2015a). On the empirical side, we find many strong sparse predictors, including price, equivalized volume, promotion, flavor scent, and brand effects. Benchmark methods including linear regression, ridge, lasso and elastic net, are used in an out-of-sample forecasting study. In particular, alpha-norm regularization provides accurate estimates for the promotion effects. Finally, we conclude with directions for future research.
REVISION: Sparse Regularization in Marketing and Economics
Date Posted:Sun, 20 Aug 2017 08:39:22 -0500
Sparse alpha-norm regularization has many data-rich applications in marketing and economics. In contrast to traditional lasso and ridge regularization, the alpha-norm penalty has the property of jumping to a sparse solution. This is an attractive feature for ultra high-dimensional problems that occur in market demand estimation and forecasting. The underlying nonconvex regularization problem is solved via coordinate descent, and a proximal operator. To illustrate our methodology, we study a classic demand forecasting problem of Bajari, Nekipelov, Ryan, and Yang (2015a). On the empirical side, we find many strong sparse predictors, including price, equivalized volume, promotion, flavor scent, and brand effects. Benchmark methods including linear regression, ridge, lasso and elastic net, are used in an out-of-sample forecasting study. In particular, alpha-norm regularization provides accurate estimates for the promotion effects. Finally, we conclude with directions for future research.
REVISION: Why Indexing Works
Date Posted:Thu, 11 May 2017 23:01:24 -0500
We develop a simple stock selection model to explain why active equity managers tend to underperform a benchmark index. We motivate our model with the empirical observation that the best performing stocks in a broad market index often perform much better than the other stocks in the index. Randomly selecting a subset of securities from the index may dramatically increase the chance of underperforming the index. The relative likelihood of underperformance by investors choosing active management likely is much more important than the loss to those same investors from the higher fees for active management relative to passive index investing. Thus, active management may be even more challenging than previously believed, and the stakes for finding the best active managers may be larger than previously assumed.
REVISION: Why Indexing Works
Date Posted:Thu, 02 Feb 2017 22:39:47 -0600
We develop a simple stock selection model to explain why active equity managers tend to underperform a benchmark index. We motivate our model with the empirical observation that the best performing stocks in a broad market index often perform much better than the other stocks in the index. Randomly selecting a subset of securities from the index may dramatically increase the chance of underperforming the index. The relative likelihood of underperformance by investors choosing active management likely is much more important than the loss to those same investors from the higher fees for active management relative to passive index investing. Thus, active management may be even more challenging than previously believed, and the stakes for finding the best active managers may be larger than previously assumed.
REVISION: Why Indexing Works
Date Posted:Sat, 26 Nov 2016 03:17:03 -0600
We develop a simple stock selection model to explain why active equity managers tend to underperform a benchmark index. We motivate our model with the empirical observation that the best performing stocks in a broad market index perform much better than the other stocks in the index. While randomly selecting a subset of securities from the index increases the chance of outperforming the index, it also increases the chance of underperforming the index, with the frequency of underperformance being larger than the frequency of overperformance. The relative likelihood of underperformance by investors choosing active management likely is much more important than the loss to those same investors of the higher fees for active management relative to passive index investing. Thus, the stakes for finding the best active managers may be larger than previously assumed.
REVISION: Deep Learning for Finance: Deep Portfolios
Date Posted:Wed, 14 Sep 2016 05:09:20 -0500
We explore the use of deep learning hierarchical models for problems in financial prediction and classification. Financial prediction problems – such as those presented in designing and pricing securities, constructing portfolios, and risk management – often involve large data sets with complex data interactions that currently are difficult or impossible to specify in a full economic model. Applying deep learning methods to these problems can produce more useful results than standard methods in finance. In particular, deep learning can detect and exploit interactions in the data that are, at least currently, invisible to any existing financial economic theory.
REVISION: Why Indexing Works
Date Posted:Thu, 15 Oct 2015 04:53:11 -0500
We develop a simple stock selection model to explain why active equity managers tend to underperform a benchmark index. We motivate our model with the empirical observation that the best performing stocks in a broad market index perform much better than the other stocks in the index. While randomly selecting a subset of securities from the index increases the chance of outperforming the index, it also increases the chance of underperforming the index, with the frequency of underperformance being larger than the frequency of overperformance. The relative likelihood of underperformance by investors choosing active management likely is much more important than the loss to those same investors of the higher fees for active management relative to passive index investing. Thus, the stakes for finding the best active managers may be larger than previously assumed.
REVISION: Why Indexing Works
Date Posted:Wed, 14 Oct 2015 01:31:28 -0500
We develop a simple stock selection model to explain why active equity managers tend to underperform a benchmark index. We motivate our model with the empirical observation that the best performing stocks in a broad market index perform much better than the other stocks in the index. While randomly selecting a subset of securities from the index increases the chance of outperforming the index, it also increases the chance of underperforming the index, with the frequency of underperformance being larger than the frequency of overperformance. The relative likelihood of underperformance by investors choosing active management likely is much more important than the loss to those same investors of the higher fees for active management relative to passive index investing. Thus, the stakes for finding the best active managers may be larger than previously assumed.
REVISION: Sequential Learning, Predictability, and Optimal Portfolio Returns
Date Posted:Thu, 04 Apr 2013 15:02:44 -0500
This paper finds statistically and economically significant out-of-sample portfolio benefits for an investor who uses models of return predictability when forming optimal portfolios. The key is that investors must incorporate an ensemble of important features into their optimal portfolio problem, including time-varying volatility, and time-varying expected returns driven by improved predictors such as measures of yield that include share repurchase and issuance in addition to cash payouts ...
REVISION: Sequential Learning, Predictability, and Optimal Portfolio Returns
Date Posted:Thu, 07 Mar 2013 12:48:00 -0600
This paper finds statistically and economically significant out-of-sample portfolio benefits for an investor who uses models of return predictability when forming optimal portfolios. The key is that investors must incorporate an ensemble of important features into their optimal portfolio problem, including time-varying volatility, and time-varying expected returns driven by improved predictors such as measures of yield that include share repurchase and issuance in addition to cash payouts ...
REVISION: Sequential Learning, Predictive Regressions, and Optimal Portfolio Returns
Date Posted:Tue, 14 Feb 2012 05:47:58 -0600
This paper finds statistically and economically significant out-of-sample portfolio benefits for an investor who uses models of return predictability when forming optimal portfolios. The key is that investors must incorporate an ensemble of important features into their optimal portfolio problem including time-varying volatility and time-varying expected returns driven by improved predictors such as measures of yield that include shares repurchase and issuance in addition to cash payouts ...
REVISION: Particle Learning and Smoothing
Date Posted:Wed, 09 Nov 2011 00:40:35 -0600
Particle learning (PL) provides state filtering, sequential parameter learning and smoothing in a general class of state space models. Our approach extends existing particle methods by incorporating the estimation of static parameters via a fully-adapted filter that utilizes conditional sufficient statistics for parameters and/or states as particles. State smoothing in the presence of parameter uncertainty is also solved as a by-product of PL. In a number of examples, we show that PL ...
REVISION: Particle Learning and Smoothing
Date Posted:Sat, 22 Oct 2011 10:11:45 -0500
Particle learning (PL) provides state filtering, sequential parameter learning and smoothing in a general class of state space models. Our approach extends existing particle methods by incorporating the estimation of static parameters via a fully-adapted filter that utilizes conditional sufficient statistics for parameters and/or states as particles. State smoothing in the presence of parameter uncertainty is also solved as a by-product of PL. In a number of examples, we show that PL ...
REVISION: Tracking Flu Epidemics Using Google Flu Trends and Particle Learning
Date Posted:Tue, 16 Mar 2010 17:49:08 -0500
In the second half of 2009 the world experienced an intense influenza activity. The new 2009 H1N1 virus, formerly known as the swine flu, has in only five months found its way from Mexico to a majority of the countries on the planet. The fears of a large second-wave pandemic and its potential impact on health and economic outcomes have underlined the importance of accurate and fast disease surveillance mechanisms capable of suggesting timely public health interventions.
In this paper we ...
REVISION: Tracking Flu Epidemics Using Google Flu Trends and Particle Learning
Date Posted:Tue, 01 Dec 2009 09:56:46 -0600
In the summer of 2009 the world experienced an intense influenza activity. The new 2009 H1N1 virus, formerly known as the swine flu, has in only five months found its way from Mexico to a majority of the countries on the planet. The fears of a large second-wave pandemic and its potential impact on health and economic outcomes have underlined the importance of accurate and fast disease surveillance mechanisms capable of suggesting timely public health interventions.
In this paper we introduce ...
REVISION: Corporate Credit Spreads under Parameter Uncertainty
Date Posted:Mon, 30 Nov 2009 10:17:45 -0600
This paper assesses the impact of parameter uncertainty on corporate bond
credit spreads. Using data for 5,300 firm-years between 1994 and 2008, we
find that investors’ uncertainty about model parameters explains up to 40% of
the credit spread that is typically attributed to liquidity, taxes and jump risk,
without significantly raising bankruptcy probabilities. Spreads on firms with
large intangible assets and volatile earnings growth are the most affected by
parameter uncertainty. Uncertainty ...
REVISION: Tracking Flu Epidemics Using Google Flu Trends and Particle Learning
Date Posted:Fri, 27 Nov 2009 09:16:34 -0600
In the summer of 2009 the world experienced an intense influenza activity. The new 2009 H1N1 virus, formerly known as the swine flu, has in only five months found its way from Mexico to a majority of the countries on the planet. The fears of a large second-wave pandemic and its potential impact on health and economic outcomes have underlined the importance of accurate and fast disease surveillance mechanisms capable of suggesting timely public health interventions.
In this paper we introduce ...
New: Sequential Inference for Nonlinear Models using Slice Variables
Date Posted:Sat, 21 Nov 2009 07:12:58 -0600
This paper develops particle-based methods for sequential inference in nonlinear models. Sequential inference is notoriously difficult in nonlinear state space models. To overcome this, we use auxiliary state variables to slice out nonlinearities where appropriate. This induces a Fixed-dimension conditional sufficient statistics and, given these, we adapt existing particle learning algorithms to update posterior beliefs about states and parameters. We provide three illustrations. First, a ...
New: Quantile Filtering and Learning
Date Posted:Sat, 21 Nov 2009 07:04:58 -0600
Quantile and least-absolute deviations (LAD) methods are popular robust statistical methods but have not generally been applied to state filtering and sequential parameter learning. This paper introduces robust state space models whose error structure coincides with quantile estimation criterion, with LAD a special case. We develop an efficient particle based method for sequential state and parameter inference. Existing approaches focus solely on the problem of state filtering, conditional on ...
New: Optimal Filtering of Jump Diffusions: Extracting Latent States from Asset Prices
Date Posted:Sat, 26 Sep 2009 17:03:07 -0500
This paper provides an optimal filtering methodology in discretely observed continuous-time jump-diffusion models. Although the filtering problem has received little attention, it is useful for estimating latent states, forecasting volatility and returns, computing model diagnostics such as likelihood ratios, and parameter estimation. Our approach combines time-discretization schemes with Monte Carlo methods. It is quite general, applying in nonlinear and multivariate jump-diffusion models and ...
REVISION: Volatility, Liquidity, Credit Spreads and Bankruptcy Prediction
Date Posted:Fri, 26 Dec 2008 10:01:32 -0600
We assess the impact of parameter uncertainty on corporate bond credit spreads. Using data for 4,206 firm-years between 1994 and 2004, we find that investors' uncertainty about the true model parameters explains up to half the credit spread that is typically attributed to liquidity, taxes and jump risk. Spreads on young and small firms with large intangible assets and volatile earnings growth are the most affected by parameter uncertainty. We find that even AAA-rated firms have positive ...
REVISION: Sequential Learning, Predictive Regressions, and Optimal Portfolio Returns
Date Posted:Sat, 04 Oct 2008 21:02:59 -0500
This paper develops particle filtering and learning for sequential inference in empirical asset pricing models. We provide a computationally feasible toolset for sequential parameter learning, hypothesis testing, and model monitoring, incorporating multiple observable variables, unobserved stochastic volatility, and unobserved "drifting" regression coefficients. Sequential inference allows us to observe how the views of economic decision makers evolve in real time. Empirically, we analyze time ...
REVISION: Volatility, Liquidity, Credit Spreads and Bankruptcy Prediction
Date Posted:Wed, 26 Mar 2008 10:33:46 -0500
We develop a methodology for estimating structural models of the firm using both debt and equity prices. Our approach uncovers market beliefs about firm value and volatility together with the liquidity discount in bond prices. We illustrate our methodology using a large panel dataset of 3,632 firm-years between 1994 and 2004. Default spreads and bankruptcy probabilities are economically different from standard calibration methods. Most importantly, allowing for parameter uncertainty explains ...
REVISION: Sequential Learning, Predictive Regressions, and Optimal Portfolio Returns
Date Posted:Tue, 25 Mar 2008 10:59:21 -0500
This paper develops particle filtering tools for sequential inference in empirical asset pricing models, providing a computationally feasible approach for sequential parameter learning, hypothesis testing, and model monitoring. The class of models incorporates multiple observable variables, unobserved stochastic volatility, and unobserved "drifting" regression coefficients. The sequential nature focuses on how the views of economic decision makers evolve in real time. Empirically, we analyze ...
New: Particle Filtering and Parameter Learning
Date Posted:Wed, 02 May 2007 11:31:56 -0500
In this paper, we provide an exact particle filtering and parameter learning algorithm. Our approach exactly samples from a particle approximation to the joint posterior distribution of both parameters and latent states, thus avoiding the use of and the degeneracies inherent to sequential importance sampling. Exact particle filtering algorithms for pure state filtering are also provided. We illustrate the efficiency of our approach by sequentially learning parameters and filtering states in ...
REVISION: Volatility, Liquidity, Credit Spreads and Bankruptcy Prediction
Date Posted:Mon, 20 Nov 2006 19:40:22 -0600
We develop a methodology for estimating structural models of the firm using both debt and equity prices. Our approach uncovers market beliefs about firm value and volatility together with the liquidity discount in bond prices. We illustrate our methodology using a large panel dataset of 3,632 firm-years between 1994 and 2004. Default spreads and bankruptcy probabilities are economically different from standard calibration methods. Most importantly, allowing for parameter uncertainty explains ...
REVISION: The Impact of Parameter Uncertainty on Credit Spreads
Date Posted:Tue, 21 Feb 2006 07:26:35 -0600
In this paper we develop a methodology for estimating structural asset pricing models where both debt and equity prices are observed over time. Our approach allows for nonlinear debt pricing models driven by unobserved state variables such as firm value. Given observed debt, equity and derivative prices, we uncover market beliefs about state variables and parameters. By incorporating parameter uncertainty in the default boundary and recovery rates we find debt pricing and probabilities of ...
MCMC Methods for Continuous-Time Financial Econometrics
Date Posted:Fri, 26 Dec 2003 14:34:33 -0600
This chapter develops Markov Chain Monte Carlo (MCMC) methods for Bayesian inference in continuous-time asset pricing models. The Bayesian solution to the inference problem is the distribution of parameters and latent variables conditional on observed data, and MCMC methods provide a tool for exploring these high-dimensional, complex distributions. We first provide a description of the foundations and mechanics of MCMC algorithms. This includes a discussion of the Clifford-Hammersley theorem, ...
The Impact of Jumps in Volatility and Returns
Date Posted:Sun, 31 Aug 2003 03:00:09 -0500
This paper examines continuous-time stochastic volatility models incorporating jumps in returns and volatility. We develop a likelihood-based estimation strategy and provide estimates of parameters, spot volatility, jump times, and jump sizes using S&P 500 and Nasdaq 100 index returns. Estimates of jump times, jump sizes, and volatility are particularly useful for identifying the effects of these factors during periods of market stress, such as those in 1987, 1997, and 1998. Using formal and ...
Nonlinear Filtering of Stochastic Differential Equations with Jumps
Date Posted:Wed, 12 Feb 2003 23:39:51 -0600
In this paper, we develop an approach for filtering state variables in the setting of continuous-time jump-diffusion models. Our method computes the filtering distribution of latent state variables conditional only on discretely observed observations in a manner consistent with the underlying continuous-time process. The algorithm is a combination of particle filtering methods and the "filling-in-the-missing-data" estimators which have recently become popular. We provide simulation evidence to ...
Sequential Optimal Portfolio Performance: Market and Volatility Timing
Date Posted:Thu, 02 May 2002 02:52:15 -0500
This paper studies the economic benefits of return predictability by analyzing the impact of market and volatility timing on the performance of optimal portfolio rules. Using a model with time-varying expected returns and volatility, we form optimal portfolios sequentially and generate out-of-sample portfolio returns. We are careful to account for estimation risk and parameter learning. Using S&P 500 index data from 1980-2000, we find that a strategy based solely on volatility timing uniformly ...
Bayesian Analysis of a Stochastic Volatility Model with Leverage Effect and Fat Tails
Date Posted:Wed, 19 Dec 2001 02:39:34 -0600
The basic univariate stochastic volatility model specifies that conditional volatility follows a log-normal auto-regressive model with innovations assumed to be independent of the innovations in the conditional mean equation. Since the introduction of practical methods for inference in the basic volatility model (JPR-(1994)), it has been observed that the basic model is too restrictive for many financial series. We extend the basic SVOL to allow for a so-called "Leverage effect" via ...
The Impact of Jumps in Volatility and Returns
Date Posted:Mon, 01 Jan 2001 05:13:44 -0600
This paper examines a class of continuous-time models that incorporate jumps in returns and volatility, in addition to diffusive stochastic volatility. We develop a likelihood-based estimation strategy and provide estimates of model parameters, spot volatility, jump times and jump sizes using both S&P 500
and Nasdaq 100 index returns. Estimates of jumps times, jump sizes and volatility are particularly useful for disentangling the dynamic effects of these factors during periods of market ...
A deep-learning model can automate successful portfolio management.
{PubDate}It’s possible to use real-time market odds data for soccer matches to determine probabilities for various margins of victory.
{PubDate}One of the biggest threats to global health is not the cost of medical care or the challenge of an aging population. It’s the flu—a pandemic-in-waiting that can go easily undetected.
{PubDate}