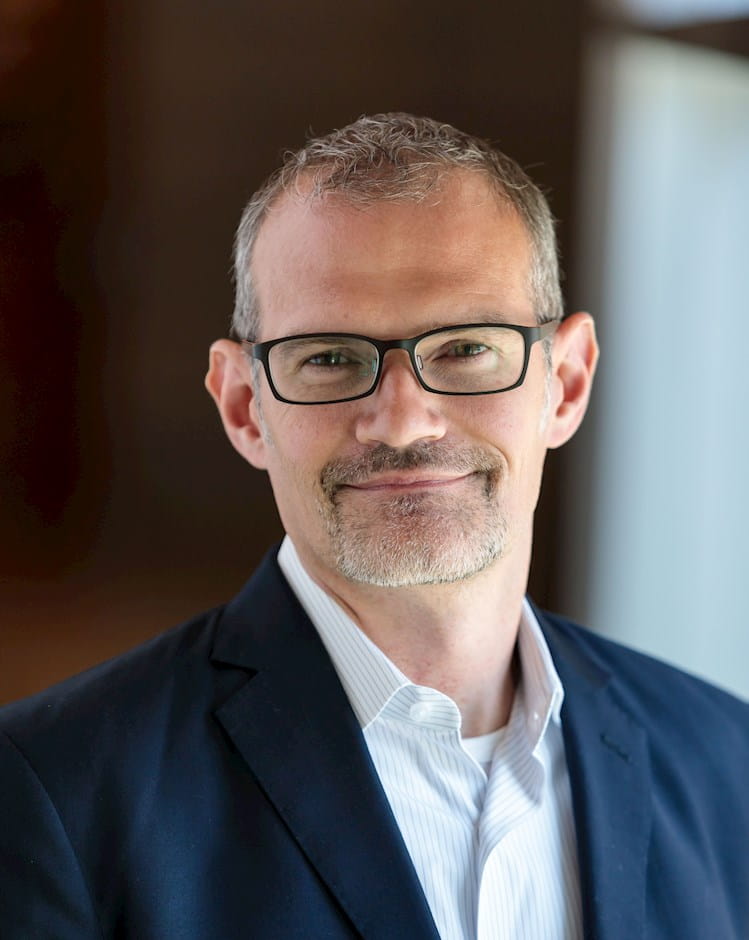
Christian B. Hansen
Wallace W. Booth Professor of Econometrics and Statistics
Wallace W. Booth Professor of Econometrics and Statistics
Christian B. Hansen is the Wallace W. Booth Professor of Econometrics and Statistics at the University of Chicago Booth School of Business. Prof. Hansen is the current academic coordinator for the Booth Executive MBA program. He is also co-editor of the Journal of Political Economy - Microeconomics.
Prof. Hansen studies applied and theoretical econometrics. His research has chiefly been in the areas of the use of high-dimensional statistical methods in economic applications, estimation of panel data models, inference using clustered standard errors, quantile regression, and weak instruments. His most recent research has looked at the use of machine learning and artificial intelligence as in input to estimation of causal and policy effects. Hansen’s published work appears in leading journals in economics and statistics including the American Economic Review, Annals of Statistics, Econometrica, Journal of Business and Economic Statistics, Journal of Econometrics, Review of Economics and Statistics, and Review of Economic Studies. Hansen has been a Neubauer Family Faculty Fellow at Booth and an NSF research grant recipient.
Hansen graduated from Brigham Young University with a bachelor's degree in economics in 2000. In 2004, he received a PhD in economics from the Massachusetts Institute of Technology, where he was a graduate research fellow of the National Science Foundation. He joined the Chicago Booth faculty in 2004.