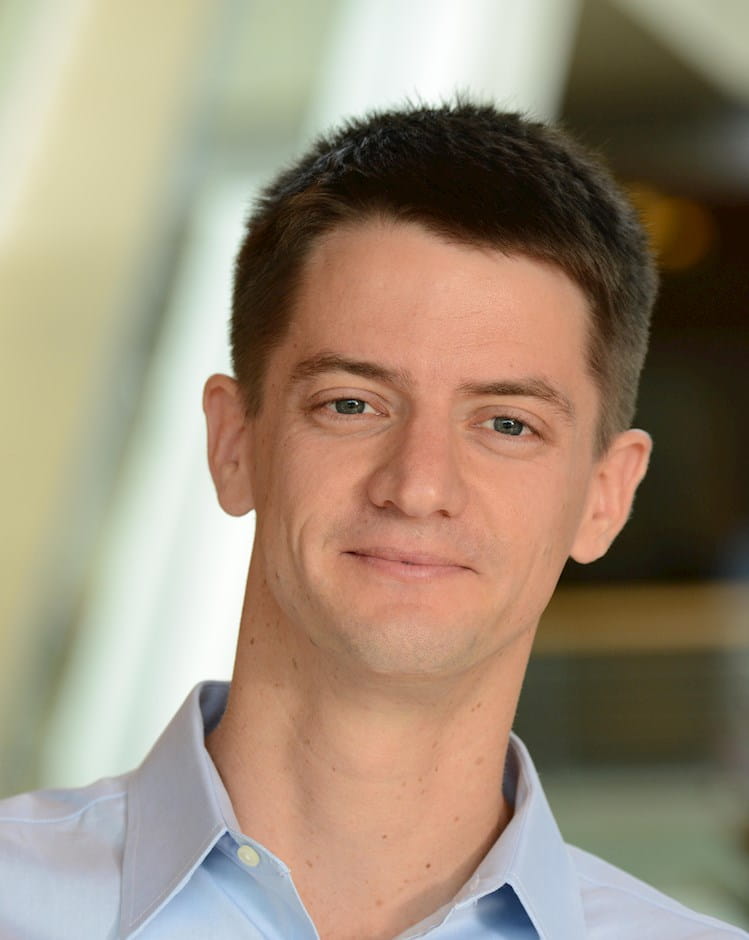
Max Farrell
Visiting Associate Professor of Economics
Visiting Associate Professor of Economics
Max H. Farrell is an Associate Professor at the University of Chicago Booth School. His research spans machine learning, high-dimensional data, and robust semiparametric and nonparametric methods, focusing on mathematical and statistical problems. Recent work has studied deep learning and its applications in economics and business applications. His research includes developing new methodology as well as increasing reliability and implementability in real-world social science applications, with an emphasis on causal inference and program evaluation. His novel analysis methods have been developed into award-winning open source software. His work appears in Econometrica, the Journal of the American Statistical Association, the Annals of Statistics, the Review of Economics and Statistics, and other outlets. He currently serves as associate editor for the Review of Economics and Statistics and on the editorial board for the Journal of Machine Learning Research.
Farrell earned a Ph.D. in economics from the University of Michigan, as well as an M.A. in statistics. Farrell pursued undergraduate studies at the Massachusetts Institute of Technology where he earned S.B. degrees in mathematics and economics. He has experience teaching statistics and econometrics at the undergraduate, masters, and graduate level.
Prior to his graduate studies, Farrell worked at the Center for Research on Health Care at the University of Pittsburgh and at Analysis Group, Inc, in Boston, where he worked on a variety of statistical, economic, and litigation consulting projects.
Number | Course Title | Quarter |
---|---|---|
41917 | Causal Machine Learning | 2023 (Autumn) |
A transformative tool is being used to answer an ever-increasing number of questions.
{PubDate}Machine learning is being tasked with an increasing number of important decisions. But the answers it generates involve a degree of uncertainty.
{PubDate}Evaluating the performance of machine-learning tools isn’t always easily done.
{PubDate}