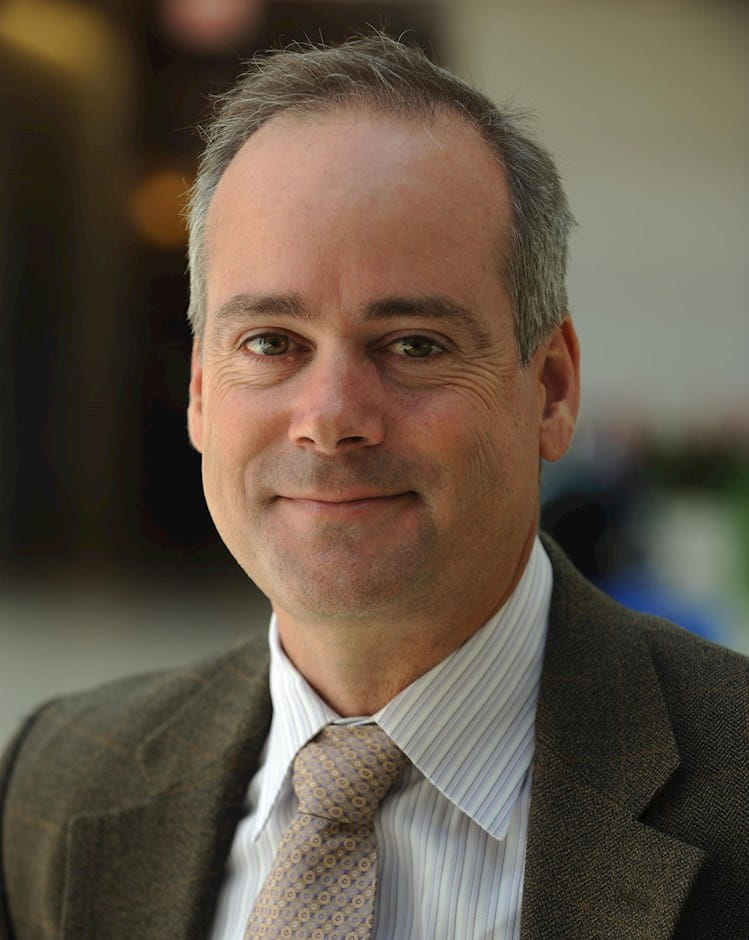
Jeffrey R. Russell
Alper Family Professor of Econometrics and Statistics
Alper Family Professor of Econometrics and Statistics
Jeffrey Russell conducts research on financial econometrics, time series, applied econometrics, empirical market microstructure, and high-frequency financial data. Russell's recent research has focused on using intraday price data to measure and predict financial asset volatility. His work has appeared in the Review of Economic Studies, Journal of Financial Economics and Econometrica. His research is supported by a Morgan Stanley Equity Microstructure Grant and he is the recipient of an Alfred P. Sloan Doctoral Dissertation Fellowship.
In addition to teaching and research, Russell is an associate editor of the Journal of Business and Economic Statistics. He has served on the NASDAQ Board of Economic Advisors. Additionally, he works as a consultant for legal and financial companies. He also has worked as a consultant for Citadel, where he modeled and forecasted intraday financial returns.
Russell has made presentations all over the world, including the 2006 North American Econometric Society Meetings, the 2005 Financial Econometrics Conference in Montreal, the 2005 Morgan Stanley Equity Microstructure Conference in Miami, and the 2005 American Finance Association Annual Meetings in Philadelphia.
He was the 2005-2006 Morgan Stanley visiting researcher at NYU. He earned a bachelor's of arts degree and a bachelor's of science degree in 1991. In 1996 he received a PhD in economics from the University of California at San Diego, where he earned an Econometric Analysis Fellowship and an Academic Excellence Award. He joined the Chicago Booth faculty in 1996.
With F. Bandi, "Microstructure Noise, Realized Volatility, and Optimal Sampling," Review of Economic Studies (forthcoming).
With F. Bandi and J. Zhu, "Using High-Frequency Data in Dynamic Portfolio Choice" Econometric Reviews (forthcoming).
With F. Bandi, "Separating Microstructure Noise from Volatility," Journal of Financial Economics (2006).
With R. Engle, "A Discrete-State, Continuous-Time Model for Financial Transactions Prices and Times: The ACM-ACD Model," Journal of Business Economics and Statistics (2006).
With R. Engle, "Autoregressive Conditional Duration: A New Model for Irregularly Spaced Transaction Data," Econometrica (1998).
For a listing of research publications, please visit the university library listing page.
New: Measuring and Modeling Execution Cost and Risk
Date Posted:Mon, 29 Dec 2008 02:18:21 -0600
We introduce a new analysis of transaction costs that explicitly recognizes the importance of the timing of execution in assessing transaction costs. Time induces a risk/cost tradeoff. The price of immediacy results in higher costs for quickly executed orders while more gradual trading results in higher risk since the value of the asset can vary more over longer periods of time. We use a novel data set that allows a sequence of transactions to be associated with individual orders and measure ...
New: Measuring and Modeling Execution Cost and Risk
Date Posted:Mon, 29 Dec 2008 02:17:44 -0600
We introduce a new analysis of transaction costs that explicitly recognizes the importance of the timing of execution in assessing transaction costs. Time induces a risk/cost tradeoff. The price of immediacy results in higher costs for quickly executed orders while more gradual trading results in higher risk since the value of the asset can vary more over longer periods of time. We use a novel data set that allows a sequence of transactions to be associated with individual orders and measure ...
Forecasting the Frequency of Changes in Quoted Foreign Exchange Prices with the Autoregressive Condi...
Date Posted:Tue, 29 Apr 2008 10:50:50 -0500
This paper applies the Autoregressive Conditional Duration model to Foreign Exchange quotes arriving on Reuter's screens. The Autoregressive Conditional Duration model, proposed in Engle and Russell (1995), is a new statistical model for the analysis of data that do not arrive in equal time intervals. When Dollar/Deutschmark data are examined, it is clear that many of the price quotes carry little information about the price process, as they are simply repeats of the previous quote. By ...
Autoregressive Conditional Duration: A New Model for Irregularly Spaced Transaction Data
Date Posted:Tue, 29 Apr 2008 10:49:51 -0500
This paper proposes a new statistical model for the analysis of data that do not arrive in equal time intervals, such as financial transactions data, telephone calls, or sales data on commodities that are tracked electronically. In contrast to fixed interval analysis, the model treats the time between events as a stochastic time varying process. We propose a new model for point processes with intertemporal correlation. Because the model focuses on the time interval between events it is called ...
Econometric Analysis of Discrete-Valued Irregularly-Spaced Financial Transactions Data Using a New A...
Date Posted:Tue, 29 Apr 2008 10:45:50 -0500
This paper proposes a new approach to modeling financial transactions data. A model for discrete valued time series is introduced in the context of generalized linear models. Since the model specifies probabilities of return outcomes conditional on both the previous state and the historic distribution, we call the it the Autoregressive Conditional Multinomial (ACM) model. Recognizing that prices are observed only at transactions, the process is interpreted as a marked point process. The ACD ...
Forecasting Transaction Rates: The Autoregressive Conditional Duration Model
Date Posted:Tue, 22 Apr 2008 07:54:04 -0500
This paper will propose a new statistical model for the analysis of data that does not arrive in equal time intervals such as financial transactions data, telephone calls, or sales data on commodities that are tracked electronically. In contrast to fixed interval analysis, the model treats the time between observation arrivals as a stochastic time varying process and therefore is in the spirit of the models of time deformation initially proposed by Tauchen and Pitts (1983), Clark (1973) and ...
Separating Microstructure Noise from Volatility
Date Posted:Sun, 02 Jan 2005 17:01:01 -0600
There are two volatility components embedded in the returns constructed using recorded stock prices: the genuine time-varying volatility of the unobservable returns that would prevail (in equilibrium) in a frictionless, full-information, economy and the variance of the equally unobservable microstructure noise. Using straightforward sample averages of high-frequency return data recorded at different frequencies, we provide a simple technique to identify both volatility features. We apply our ...