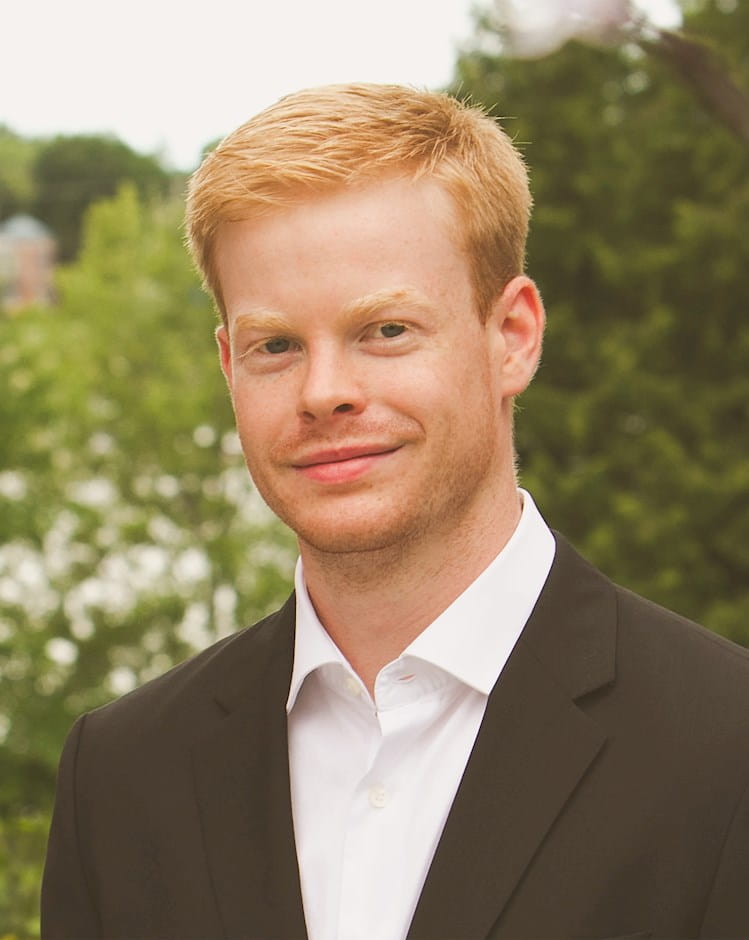
Berkeley J. Dietvorst
Associate Professor of Marketing
Associate Professor of Marketing
My research focuses on consumer and managerial decision making. Specifically, I aim to understand how people use information to make judgments and decisions, particularly in risky or uncertain domains. My main stream of research in this area investigates consumers’ and managers’ use of algorithms to make predictions. In my other research, I investigate other cases of judgment and decision making under risk or uncertainty, including: consumers’ attitudes towards corporate experiments, researchers’ use of replication to assess generalizability, consumers’ risk preferences, consumers’ prediction strategies, choice architecture, people’s ability to disregard information that has been discredited, and the consequences of performance expectations for persistence.
Number | Course Title | Quarter |
---|---|---|
37000 | Marketing Strategy | 2023 (Autumn) |
Number | Course Title | Quarter |
---|---|---|
37000 | Marketing Strategy | 2025 (Spring) |
The Chicago Booth Review Podcast explores questions about the role of A.I. in society.
{PubDate}The moral relevance of a decision affects people’s preference for who makes it.
{PubDate}Research unpacks why people often prefer the predictions of humans.
{PubDate}